Can AI be “Death Predictor” ??
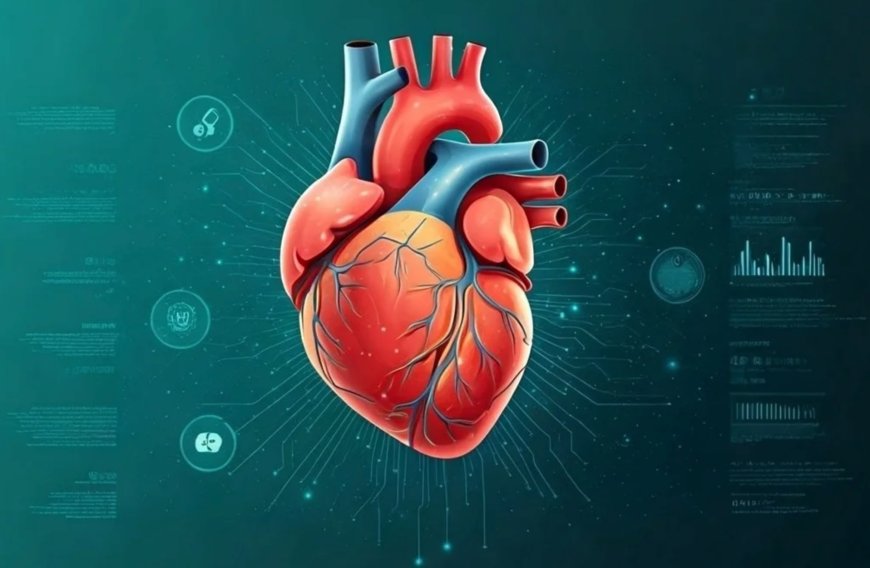
For ages, people have pondered the mysteries of mortality, striving to comprehend life while also finding ways to face death more effectively. In the last few years, the rise of Artificial Intelligence (AI) has ignited fresh inquiries into the possibility of predicting death. As healthcare expenses soar, life expectancy varies, and personalised medicine progresses, AI has emerged as a promising resource for assessing mortality risk. However, how dependable is this technology? Is it truly possible to foresee death, or are we placing too much faith in AI's capabilities?
The State of Mortality Prediction: Where We Are Today
The quest to predict death may seem like an ancient endeavour, but it is only with the advent of modern computational capabilities that we can effectively analyse intricate biological, environmental, and behavioural data on a large scale. Today, research institutions and healthcare organizations are harnessing machine learning models to accurately forecast mortality risk.
For example, a study conducted by Stanford University utilised an AI model to examine Electronic Health Record (EHR) data, achieving approximately 90% accuracy in predicting mortality within a year for hospitalised patients. Similarly, the AI team at Google Health applied deep learning techniques to evaluate hospitalisation records, generating risk predictions that can surpass those made by human doctors. IBM Watson has also made significant progress by collaborating with hospitals to refine predictive algorithms for ICU patients, where timely assessments of mortality risk are essential.
AI Techniques and Data Used in Mortality Prediction
Mortality prediction relies on vast amounts of health-related data—EHRs, genetic data, imaging scans, lifestyle metrics, and even social determinants of health like socioeconomic status. Predictive models in this area typically use:
- Supervised Machine Learning: Algorithms are trained on historical data with labeled outcomes (e.g., patient survival) to forecast future mortality.
- Natural Language Processing (NLP): NLP helps AI to interpret medical notes in EHRs, extracting unstructured data that may indicate risk factors overlooked in structured datasets.
- Deep Learning Models: Neural networks, particularly Convolutional Neural Networks (CNNs) and Recurrent Neural Networks (RNNs), are employed to detect patterns in complex data sources like CT scans or MRI imaging, identifying markers associated with terminal illnesses.
- Survival Analysis Techniques: Originally developed in actuarial science, survival analysis models like Cox proportional hazards are now enhanced by machine learning, allowing AI to better assess time-dependent mortality risks.
AI-powered predictive models analyse electronic health records (EHRs), lab results, and medical imaging to identify patterns and forecast mortality probabilities. According to a study by the Healthcare Information and Management Systems Society (HIMSS), 70% of US hospitals use predictive analytics to improve patient outcomes. This technology has the potential to reduce hospital mortality rates by 50% and identify high-risk patients, who account for 50% of healthcare costs. Several notable studies demonstrate AI's potential in mortality prediction. Google's AI-powered predictive model (2019) achieved 95% accuracy in certain cases. The University of California's AI-driven algorithm (2020) identified ICU patients at high risk of mortality with 85% accuracy. A Mayo Clinic study (2020) predicted cardiac arrest with 90% accuracy. Furthermore, a University of Chicago study found that AI-powered predictive models reduced hospital mortality rates by 15%.
Breakthroughs made and on the Horizon
AI-powered predictive models are transforming the analysis of electronic health records (EHRs), lab results, and medical imaging to identify trends and assess mortality risks. According to a report from the Healthcare Information and Management Systems Society (HIMSS), 70% of hospitals across the United States are utilising predictive analytics to improve patient care. This cutting-edge technology has the potential to reduce hospital mortality rates by 50% and identify high-risk patients, who account for half of healthcare spending. Numerous pivotal studies underscore the success of AI in mortality prediction. For example, Google's AI model from 2019 achieved an impressive 95% accuracy in certain situations. In 2020, researchers at the University of California developed an algorithm that accurately identified ICU patients at high risk of death 85% of the time. Additionally, a Mayo Clinic study from the same year predicted cardiac arrest with a 90% accuracy rate. Furthermore, research from the University of Chicago suggested that AI-driven predictive models could decrease hospital mortality rates by 15%.
Several ongoing initiatives indicate promising advancements in mortality prediction. The UK’s Biobank study is gathering extensive genetic and health data from half a million individuals to create predictive models for various diseases, including those with fatal outcomes. Meanwhile, the Mayo Clinic has harnessed AI to create a predictive model for sudden cardiac death using ECG data, achieving accuracy levels previously thought unattainable.
The AI model – known as AI-ECG risk estimation, or AIRE – was able to correctly identify the risk of death in the ten years following the ECG (from high to low) in 78% of cases.
These initiatives showcase the immense potential of AI while also highlighting that no model is without its limitations. Predicting mortality is inherently complex, involving numerous interrelated variables that are not fully understood.
Experts stress the necessity of combining AI with clinical knowledge. "AI should enhance, not replace, human decision-making," states Dr. Eric Topol, a cardiologist and author. "The future of healthcare hinges on the collaboration between humans and AI."
Industry Applications: Healthcare, Insurance, and Beyond
Healthcare: The application of AI in predicting mortality is gaining significant traction across various healthcare environments, including intensive care units and long-term care facilities. For example, AI algorithms assist physicians in identifying high-risk patients who may benefit from timely interventions. These advanced systems provide a level of accuracy that fosters a proactive approach to medical treatment, shifting the focus from reactive care.
Insurance: The landscape of life and health insurance has been revolutionised by mortality prediction, with AI models becoming essential in the underwriting process. By analysing medical histories and lifestyle choices, these models allow insurers to tailor policies, modify premiums, and enhance risk management strategies.
Elderly Care: Organizations providing elderly care are leveraging predictive analytics to keep track of the health of aging individuals, pinpointing moments when medical interventions might be necessary. This approach not only promotes better health outcomes for patients but also helps organizations manage their resources more effectively.
Challenges and Ethical Considerations
Mortality prediction holds immense potential, yet it brings forth profound ethical dilemmas. Could the act of predicting death disrupt the vital relationship between patients and their doctors? There are valid worries that awareness of their predicted mortality could have a psychological toll on both patients and caregivers. Additionally, if misapplied in areas such as employment, health insurance, or credit assessments, mortality predictions could lead to socioeconomic repercussions.
Concerns about data privacy and algorithmic bias are also critical. The fairness of AI models hinges on the quality of the data they are trained on. If health data contains socioeconomic biases, it can result in distorted predictions that unfairly impact marginalized groups. Therefore, it is crucial to establish ethical guidelines for the application of these AI-driven predictions.
Despite the progress made, numerous challenges remain. The intricacies of human biology are vast, and AI often struggles to incorporate subtle contextual elements. Issues related to data quality and bias are significant as well. Dr. Suchi Saria, CEO of Bayesian Health, emphasises that while "AI can identify patterns, context and clinical expertise are essential." Similarly, Dr. Atul Gawande, a surgeon and author, asserts that "Predictive models should enhance, not replace, human judgment."
The Future: Can AI Ever Truly Predict Mortality?
Accurately predicting death remains a challenging endeavour due to the complex interplay of genetics, lifestyle, environment, and chance. However, the influence of AI in mortality prediction is steadily increasing. In the near future, innovations in data integration, privacy-preserving methods, and federated learning could lead to more refined predictive models, enhancing their accuracy while ensuring patient confidentiality.
Instead of solely concentrating on the prediction of death, the primary objective may be to enhance the quality of life and healthcare outcomes by empowering individuals to make informed health decisions. As AI technology evolves, its function in mortality prediction could shift towards serving as a supportive tool rather than a definitive forecast, focusing on improving care, guiding choices, and fostering overall well-being.
This involves creating more sophisticated predictive models that consider contextual elements, improving data quality and diversity to minimize bias, and merging AI capabilities with clinical expertise to enhance decision-making processes.
Conclusion
The convergence of artificial intelligence and mortality forecasting offers a remarkable chance to improve sectors like healthcare, insurance, and elder care. As we move forward, there is optimism that these AI-generated insights will equip individuals and their caregivers, fostering healthier lifestyles and more responsive institutions. Although a concrete “death predictor” is still beyond our grasp, the exploration of mortality prediction highlights the potential of technology alongside the intricate, profoundly human aspects of life and death.
What's Your Reaction?
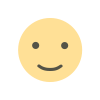

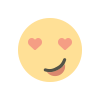



